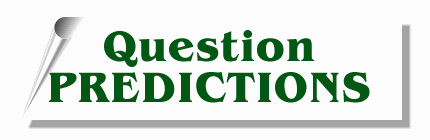
The scientists at Real Climate explain that to compare a prediction with observations one must assess whether the observations fall within a range defined as 95% of model realizations. In other words, if you run a model, or a set of models, 100 times, you would take the average of the 100 runs and plot the 95 individual runs closest to that average, and define that range as an "envelope" of projections. If observations fall within this envelope, they you would declare the projection to be a predictive success.
Imagine if a weather forecaster said that he ran a model 100 times and it showed that tomorrow's temperature was going to be between 25 and 75 degrees, with 95% confidence, with a "best estimate" of 50 degrees. If the temperature came in at 30 degrees you might compare it to the "best estimate" and say that it was a pretty poor forecast. If the weather forecast explained that the temperature was perfectly "consistent with" his forecast, you'd probably look for another forecaster. If the true uncertainty was actually between 25 and 75 degrees, then one might question the use of issuing a "best estimate."
Gavin Schmidt explains how this works in the context of the current "pause" (his words) in the increase in global average surface temperatures over the past 11 years (emphasis added):
The trend in the annual mean HadCRUT3v data from 1998-2009 (assuming the year-to-date is a good estimate of the eventual value) is 0.06+/-0.14 ºC/dec (note this is positive!). If you want a negative (albeit non-significant) trend, then you could pick 2002-2009 in the GISTEMP record which is -0.04+/-0.23 ºC/dec. The range of trends in the model simulations for these two time periods are [-0.08,0.51] and [-0.14, 0.55], and in each case there are multiple model runs that have a lower trend than observed (5 simulations in both cases). Thus ‘a model’ did show a trend consistent with the current ‘pause’. However, that these models showed it, is just coincidence and one shouldn’t assume that these models are better than the others. Had the real world ‘pause’ happened at another time, different models would have had the closest match.Think about the logic of "consistent with" as used in this context. It means that the larger the model spread, the larger the envelope of projections, and the greater the chance that whatever is observed will in fact fall within that envelope. An alert reader points this out to Gavin in the comments:
I can claim I’m very accurate because my models predict a temperature between absolute zero and the surface temperature of the sun, but that error range is so large, it means I’m not really predicting anything.Gavin says he agrees with this, which seems contrary to what he wrote in the post about 11-year trends. Elsewhere Gavin says such statistics are meaningful only for 15 years and longer. If so, then discussing them in terms of "consistency with" the model spread just illustrates how this methodology can retrieve a misleading signal from noise.
About 18 months ago I engaged in a series of exchanges with some in the climate modeling community on this same topic. The debate was frustrating because many of the climate scientists thought hat we were debating statistical methods, but from my perspective we were debating the methodology of forecast verification.
At that time I tried to illustrate the "consistent with" fallacy in the context of IPCC projections using the following graph. The blue curve shows a curve fit to 8-year surface temperature trends from 55 realizations from models used by IPCC (the fact that it was 8 years is irrelevant to this example). With the red curve I added 55 additional "realizations" produced from a random number generator. The blue dot shows the observations. Obviously, the observations are more "consistent with" the red curve than the blue curve. We can improve consistency by making worse predictions. There is obviously something wrong with this approach to comparing models and observations.

1. A specific prediction has to be identified when it is being made. A prediction in this case should be defined as the occurrence of some event in the future, that is to say, after the prediction is made. For the IPCC AR4 this might generously be defined as starting in 2001.
2. Pick a quantity to be forecast. This might be global average surface temperature as represented by GISS or CRU, the satellite lower tropospheric records, both or something else. But pick a quantity.
3. Decide in advance how you are going to define the uncertainty in your forecast. For instance, the IPCC presented an uncertainty range in its forecast in a manner differently than does Real Climate. Defining uncertainty is of critical importance.
For instance, eyeballing the Real Climate IPCC Figure one might be surprised to learn that had there been no temperature change from 1980 to 2010, this too would have been "consistent with" the model realization "envelope." While such uncertainty may in fact be an accurate representation of our knowledge of climate, it is certainly not how many climate scientists typically represent the certainty of their knowledge.
If any of 1, 2 or 3 above is allowed to vary and be selected in post-hoc fashion it sets the stage for selections of convenience that allow the evaluator to make choices that pretty much show whatever he wants to show.
4. A good place to start is simply with IPCC "best estimate" One can ask if observations fall above or below that value. Real Climate's post suggest that actual temperatures fall below that "best estimate."
5. You can then ask if falling below or above that value has any particular meaning with respect to the knowledge used to generate the forecast. To perform such an evaluation, you need a naive forecast, some baseline expectation against which you can compare your sophisticated forecast. In the case of global climate it might be a prediction of no temperature change or some linear fit to past trends. If the sophisticated method doesn't improve upon the naive baseline, you are not getting much value from that approach.
The bottom line is that with respect to #4 Real Climate shows that actual temperatures are running below a central estimate from the IPCC AR4 as well as below the various scenarios presented by Jim Hansen in 1988. What does this mean? Probably not much. But at the same time it should be obvious that this data should not be used as evidence to announce the successes of climate predictions, as Real Climate does: "the matches to observations are still pretty good, and we are getting to the point where a better winnowing of models dependent on their skill may soon be possible." Such over-hyping of the capabilities of climate science serves neither science nor policy particularly well. The reality is that while the human influence on the climate system is real and significant, predicting its effects for coming years and decades remains a speculative enterprise fraught with uncertainties and ignorance.